Model Improvements: v30
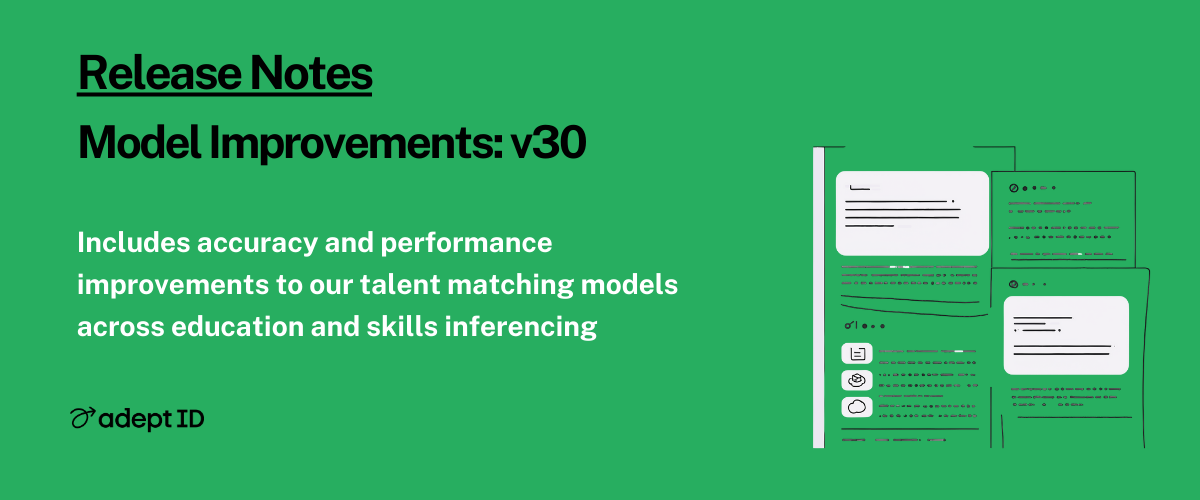
AdeptID is releasing Ensemble Model version 30 to customers! This major update brings significant improvements to our talent-matching capabilities, offering increased model accuracy and performance.
Why did we do this work? We wanted to ...
improve the way we assess fit based on education, an important part of a candidateās story. This release helps us move toward a more holistic, exhaustive view of a candidate.
increase the depth and specificity with which we understand a candidate's skills and make assessments on that basis.
better evaluate early-career candidates with little to no relevant work history, ensuring we better serve customers matching new college graduates, career changers, etc.
Bottom Line:
We've increased the number of inputs to our skill inference models by 20%, driving better alignment between our match outputs and user expectations
What are the details
-
Enhanced education matching:
We've revamped our assessment of fit based on education by ensuring our Ensemble Model includes a new and improved sub-model for education.
The sub-model takes as input a text string description of a degree program (e.g., āBachelorsā, āBachelors of Scienceā, āBachelor of Science in Nursingā) and a job title (e.g., āDialysis Nurseā) and assesses the likelihood that an individual with the stated education background might hold the specified job.
Benefits: This new sub-model enables AdeptID to more accurate matching for roles with specific educational requirements and offers a more holistic view of candidates, especially recent graduates and students with limited work experience.
-
Improved skill inferencing from job titles:
We've enhanced our ability to infer key skills directly from job titles. Job titles often communicate specific skill sets held by an individual with that title. For example, recognizing that a "Java Engineer" is proficient in Java or that a "Deep Learning Scientist" may have experience with TensorFlow.
v30 infers these key skillsets from job titles leading to improved comprehension of job and candidate skill profiles.
Benefits: Leads to a more precise understanding of candidate and job skill profiles, improving overall match quality.
See skill inferencing improvement in action!
In our demo environment we see that modifying similar job titles automatically updates the pool of candidates to reflect the subtle differences in requirements. For example:
-
Support for early-career candidates
With v30 candidates are no longer required to have work history for our models to make an assessment. They can have any combination of work experience, skills, and educationāor none at all. Candidates without detailed profiles are assumed to have baseline competencies suitable for entry-level positions that typically require limited or no prerequisites.
Benefits: Expands support to a broader talent pool, including early-career job seekers and students, allowing customers to discover promising candidates who might have previously been overlooked.